SciPy - Binomial Distribution
Binomial Distribution is a discrete probability distribution and it expresses the probability of a given number of successes in a sequence of n independent experiments with a known probability of success on each trial.
The probability mass function (pmf) of binomial distribution is defined as:
Where,
- p is the probability of success in each trial
- q is the probability of failure in each trial, q = 1 - p
- n is number of trials
- k is the number of successes which can occur anywhere among the n trials
An binomial distribution has mean np and variance npq.
The cumulative distribution function (cdf) evaluated at k, is the probability that the random variable (X) will take a value less than or equal to k. The cdf of binomial distribution is defined as:
Where, [k] is the greatest integer less than or equal to k.
The scipy.stats.binom contains all the methods required to generate and work with a binomial distribution. The most frequently methods are mentioned below:
Syntax
scipy.stats.binom.pmf(k, n, p, loc=0) scipy.stats.binom.cdf(k, n, p, loc=0) scipy.stats.binom.ppf(q, n, p, loc=0) scipy.stats.binom.rvs(n, p, loc=0, size=1)
Parameters
k |
Required. Specify float or array_like of floats representing random variable. |
q |
Required. Specify float or array_like of floats representing probabilities. |
n |
Required. Specify number of trials, must be >= 0. Floats are also accepted, but they will be truncated to integers. |
p |
Required. Specify probability of success in each trial, must be in range [0, 1]. float or array_like of floats. |
loc |
Optional. Specify the location of the distribution. Default is 0. |
size |
Optional. Specify output shape. |
binom.pmf()
The binom.pmf() function measures probability mass function (pmf) of the distribution.
from scipy.stats import binom import matplotlib.pyplot as plt import numpy as np #creating an array of values between #0 to 20 with a difference of 1 x = np.arange(0, 20, 1) y = binom.pmf(x, 20, 0.5) plt.plot(x, y, 'bo') plt.show()
The output of the above code will be:
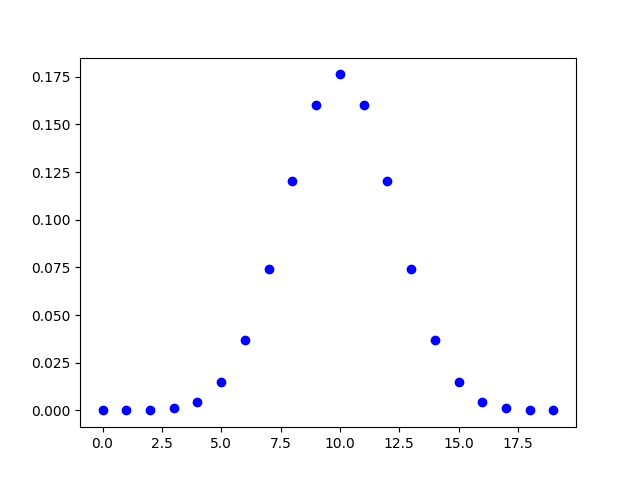
binom.cdf()
The binom.cdf() function returns cumulative distribution function (cdf) of the distribution.
from scipy.stats import binom import matplotlib.pyplot as plt import numpy as np #creating an array of values between #0 to 20 with a difference of 0.01 x = np.arange(0, 20, 0.01) y = binom.cdf(x, 20, 0.5) plt.plot(x, y) plt.show()
The output of the above code will be:
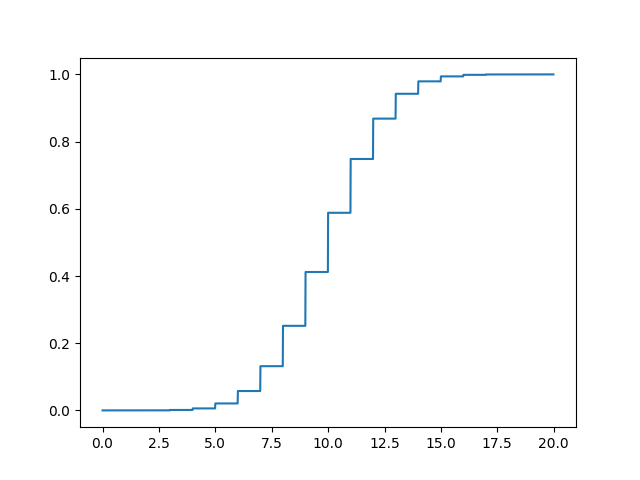
binom.ppf()
The binom.ppf() function takes the probability value and returns cumulative value corresponding to probability value of the distribution.
from scipy.stats import binom import matplotlib.pyplot as plt import numpy as np #creating an array of probability from #0 to 1 with a difference of 0.001 x = np.arange(0, 1, 0.001) y = binom.ppf(x, 20, 0.5) plt.plot(x, y) plt.show()
The output of the above code will be:
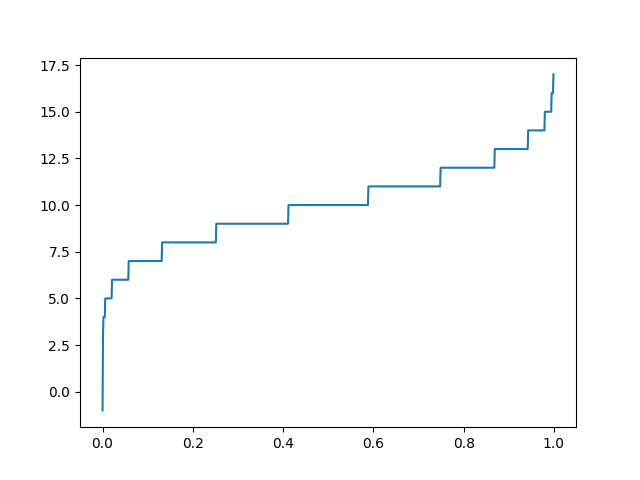
binom.rvs()
The binom.ppf() function generates an array containing specified number of random values drawn from the given binomial distribution. In the example below, a histogram is plotted to visualize the result.
from scipy.stats import binom import matplotlib.pyplot as plt import numpy as np #fixing the seed for reproducibility #of the result np.random.seed(10) #creating a vector containing 10000 #random values from binomial distribution y = binom.rvs(20, 0.5, 0, 10000) #creating bin bin = np.arange(0,25,1) plt.hist(y, bins=bin, edgecolor='blue') plt.show()
The output of the above code will be:
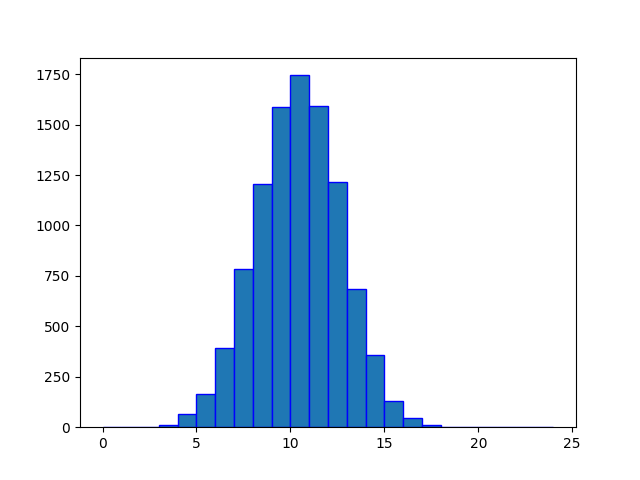